Research Article | Open Access
Insilico toxicity prediction by using ProTox-II computational tools
Sambasivam Dhinesh Kumar1, Rajasekaran. A1, K. Suresh Kumar1
1Department of Pharmaceutical Chemistry, KMCH College of Pharmacy, Coimbatore-641048, Tamil Nadu, India.
Correspondence: S. Dhinesh Kumar (Department of Pharmaceutical Chemistry, KMCH College Of Pharmacy, Affiliated to the Tamil Nadu Dr. M.G.R Medical University, Coimbatore 641048, Tamil Nadu, India; E-mail: dhineshsasi13@gmail.com).
Asia-Pacific Journal of Pharmacotherapy & Toxicology 2024, 4: 41-46. https://doi.org/10.32948/ajpt.2024.07.22
Received: 17 Mar 2024 | Accepted: 22 Jul 2024 | Published online: 29 Aug 2024
Methods ProTox-II integrates computational techniques to predict chemical toxicity endpoints, leveraging machine learning, pharmacophores, and diverse experimental data. Models are meticulously validated for accuracy on independent datasets.
Results ProTox-II's validated models ensure accurate toxicity prediction. Accessible via the web, it serves toxicologists, agencies, chemists, and stakeholders, providing comprehensive insights including toxicity radar charts, compound similarity, and detailed toxicity profiles with confidence scores.
Conclusion ProTox-II is crucial for the pharmaceutical and regulatory sectors, enhancing safety evaluations and regulatory compliance. Leveraging computational techniques, it accelerates drug discovery, serving as an essential tool for mitigating toxicity risks and advancing chemical safety assessment.
Key words pharmacophore, ProTox-II, toxicity prediction
There are various benefits when comparing the ProTox-II website to current computational models. Information on targets for both chemicals and molecules is available on the ProTox website. The ProTox-II webserver is distinct in that it classifies its prediction method according to toxicity levels, which include oral toxicity, hepatotoxicity, cytotoxicity, immunotoxicity, and mutagenicity, as well as toxicological pathways (AOPs) and toxicity targets. This classification sheds light on putative molecular pathways underlying harmful reactions. To forecast a range of toxicity endpoints, the latest version of ProTox-II combines fragment propensities, pharmacophore-based analysis, most frequent features, molecular similarity, and machine learning models. ProTox-II is a publicly accessible online server for computational toxicity prediction that offers 33 models, making it possible to predict the greatest number of toxicological endpoints to date [1].
Input Parameter: ProTox-II's interface provides simple navigation and straightforward operation. Potential toxicities linked to a chemical structure can be predicted by users by either entering the Simplified Molecular-Input Line-Entry System (SMILES) string for the compound or its name. Furthermore, users can sketch the chemical structure using the Chemdoodle chemical editor tool. Additionally, users can acquire chemical structures using compound names by utilizing the integrated PubChem search tool. Users can choose to use any of the available models or select additional models for specialized purposes such as prediction. The website automatically calculates projections for acute toxicity and toxicity targets if no further models are specified [2].
Output Parameter: The output is characterized by the instantaneous generation of data regarding acute toxicity and toxicity target forecasts. The information displayed on the result page includes the expected weight-based median fatal dose (LD50) in mg/kg, the toxicity class, the prediction accuracy, and the average similarity. The three dangerous substances that are most comparable to each other in the dataset, as determined by known rodent oral toxicity values, are also shown. Details like target names, average fit, and similarity metrics between the input compound and the pharmacophore and known ligands for each target are given when data on expected toxicity targets is available. In addition, a table with each model's confidence score and prediction results is displayed if the user chooses to select further models. When it is not possible to provide prediction results immediately, users are given a website URL to view the results. Results include a toxicity radar image that shows the average confidence score of the input compound relative to active compounds in each model's training set (Figure 1). After computation, viewers can evaluate this plot by clicking the "Open Toxicity Radar Chart" link on the result page. Users can also view a comparable chart by clicking on the thumbnail located under the Toxicity Models Report. For additional insights, an example compound output and extensive details are provided on the ProTox-II homepage [3].
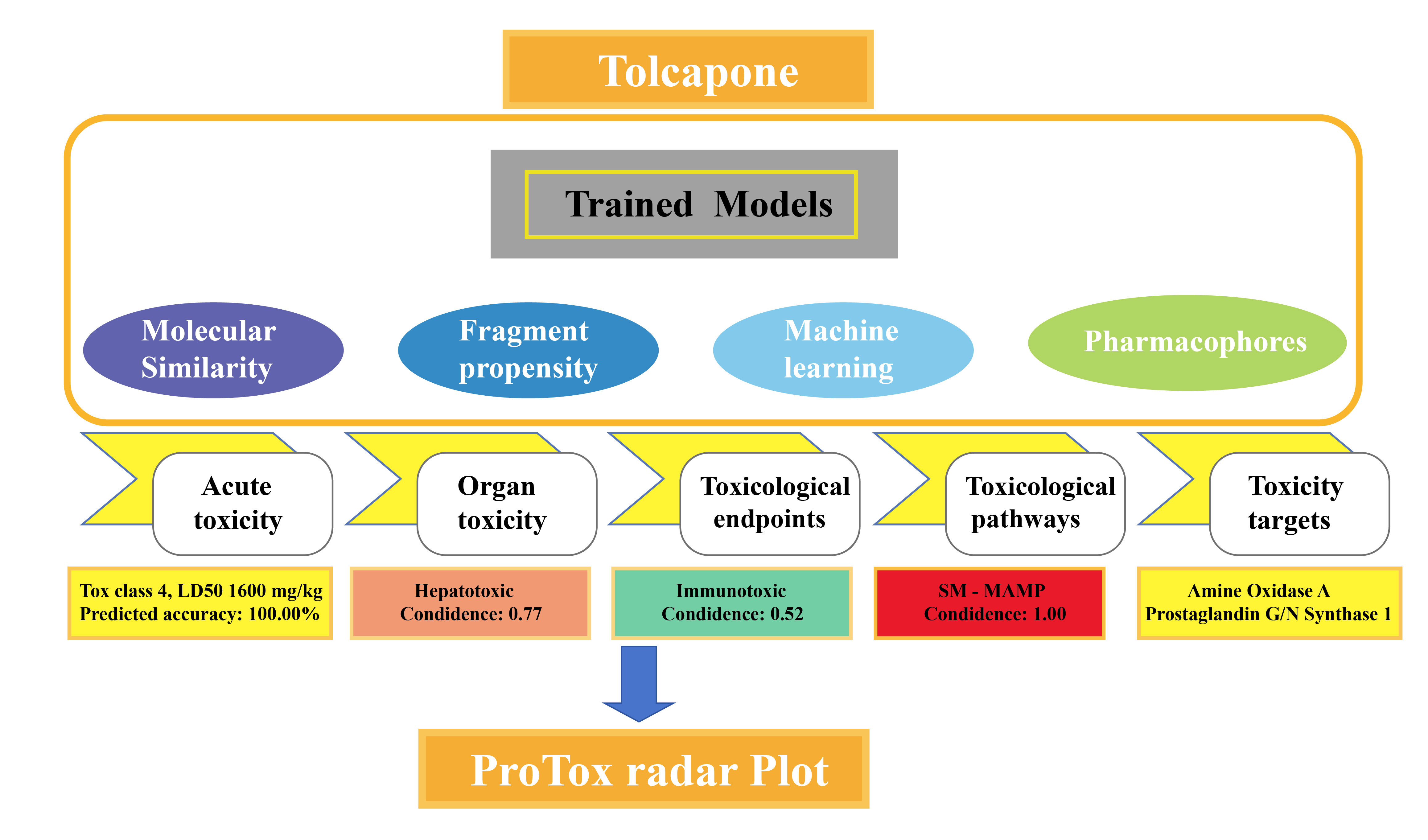
Acute toxicity
Oral toxicity: Acute toxicity models are developed based on the presence of dangerous fragments and chemical similarities between compounds with established detrimental effects.
Targets of toxicity
Toxicity targets are predicted using 15 different protein targets from in vitro safety panels linked to adverse drug responses.
Endocrine toxicology
One of the main reasons for sudden liver failure is hepatotoxicity, and drug-induced hepatotoxicity is a key reason why drugs are taken off the market. DILI, or drug-induced liver injury, can happen infrequently or over time. The prediction of DILI is still seen as a critical and safety concern by regulators, doctors, and pharmaceutical companies. Data utilized for the DILI prediction come from the NIH Liver Tox database and DILI rank. Utilizing an external validation approach with 86.00% balanced accuracy and a cross-validation accuracy of 82.00% 86.00% balanced accuracy on the external validation technique and balanced accuracy on cross-validation [6].
Resultant toxicity
ProTox-II is a webserver that predicts the toxicity of chemical compounds using machine learning algorithms. Users input chemical structures, and the platform analyzes them based on extensive datasets to assess key toxicity endpoints, including acute toxicity (LD50 values), organ toxicity (hepatotoxicity, nephrotoxicity, cardiotoxicity, and neurotoxicity), genotoxicity, carcinogenicity, endocrine disruption, and food allergy prediction. It combines these predictions into a comprehensive toxicity profile, detailing the toxic dose, affected organs, and health risks. Results are presented in a user-friendly format with visual aids, and detailed reports can be generated. Regular updates with new data and models maintain the platform's accuracy and reliability, continuously integrating new endpoints to enhance its capabilities, supporting safer drug development and chemical testing.
Carcinogenicity
Substances classified as carcinogens can either induce or increase the incidence of cancers. The Carcinogenic Potency Database (CPDB) and the CEBS database provide information for predicting carcinogenicity. The ProTox-II carcinogenicity prediction model performs well, with 81.24% balanced accuracy on cross-validation and 83.30% balanced accuracy on external validation. AUC-ROC values for cross-validation and external validation are 0.85.
Mutagenicity
The capacity to result in genetic changes Mutagens are chemicals that cause abnormal genetic mutations, like changes to a cell's DNA. These changes can harm cells and cause certain illnesses, such as cancer. The CEBS database and the benchmark data set for the Adams test are the sources of ProTox-II mutagenicity prediction. The ProTox-II mutagenicity prediction model works well, with 84.00% balanced accuracy on cross-validation and 85.00% balanced accuracy on external validation. AUC-ROC values for cross-validation and external validation are 0.90 and 0.91, respectively [7].
Cytotoxicity
Predicting a substance's potential to cause either desired or undesirable cell damage the latter being the case with tumor cells is essential for screening compounds. The ProTox-II cytotoxicity model was created using data extracted from the Chemical European Biology Laboratory (ChEMBL) database. In vitro toxicity tests on HepG2 cells, compounds with an IC50 value of less than or equal to 10 μm are considered positively cytotoxic. The ProTox-II cytotoxicity prediction model performs well, with 83.60% balanced accuracy on external validation and 85.00% balanced accuracy on cross-validation. AUC-ROC ratings for cross-validation and external validation are 0.89 and 0.90, respectively [8].
Immunotoxicity
The detrimental effects of xenobiotics on the immune system are referred to as immunotoxicity. The National Cancer Institute (NCI) in the United States provided the immune cell cytotoxicity data that were used in the immunotoxicity model. GI50 values, which are derived from the growth inhibition of the B-cell line RPMI-8226, are used to classify compounds as dangerous if they are less than 10 μm. The accuracy of the ProTox-II immunotoxicity prediction model is 70.00% in external validation and 74.00% in cross-validation. AUC-ROC values for cross-validation and external validation are 0.76 and 0.74, respectively.
Toxicological pathways
Toxicology in the 21st Century (Tox21), a US toxicology initiative, was introduced in 2008. It provides a high-throughput test library of 10,000 chemical data that has been evaluated against a panel of 12 distinct biological target-based pathways, including the two primary types of adverse outcome pathways (AOPs), the nuclear receptor pathway and the stress response pathway.
ProTox-II makes predictions about which chemicals are active in toxicological pathways based on the Tox21 dataset [9].
Nuclear receptor signaling pathways
There are seven target-pathway-based models under nuclear receptor signaling pathways: aryl hydrogen receptor (AhR), androgen receptor (AR), androgen receptor ligand binding domain (AR-LBD), aromatase, estrogen receptor alpha (ER), estrogen receptor ligand binding domain (ER-LBD), and peroxisome proliferator-activated receptor gamma (PPAR-Gamma). For both external validation and cross-validation, the models39; AUC-ROC values fall between 0.75 and 0.90, and their balanced accuracy exceeds 80%.
Five target-pathway-based models under stress response pathways represent the routes involved in the stress response: The phosphoprotein tumor suppressor (p53), heat shock factor response element (HSE), nuclear factor (erythroid-derived 2)-like 2/antioxidant responsive element (ARE), Among the proteins involved in this process are ATPase family AAA domain-containing protein 5 (ATAD5) and mitochondrial membrane potential (MMP). Each model has an AUC-ROC value between 0.80 and 0.90 for both cross-validation and external validation, and an accuracy balance exceeds 80%.
Here, two different fingerprints are used: the 166-bit MACCS molecular fingerprints and the Morgan circular fingerprinting (2048 bits) (http://www.rdkit.org/). These two fingerprints are the most effective at predicting chemical activity, according to 11 and 24.
A selective oversampling of the minority class is also included in the model-building process. The fragmentation methods of ROTBONDS and RECAP are used to separate the active (positive) and inactive (negative) data for each of are re-separated for every prediction endpoint using the fragmentation methods of ROTBONDS and RECAP. The propensity score (PS) is computed for each uniquely occurring fragment in both sets. Only molecules with the highest propensity scores for conserved fragments for the active class are oversampled and included in the model-building process. For each cross-validation fold, the ratio of active to inactive compounds was maintained constant using the fragment-based similarities between the compounds [10].
b) Validation Results: Every new model is validated using fragment-based Cluster 10-fold cross-validation. Ten sets of data were created using a fragment similarity-based sampling strategy, nine of which were used to train the model and the tenth to validate it while keeping constant ratios of active to inactive. In addition, outside datasets that weren't part of the training set were used to externally validate the models. The following performance measures are used to assess the models: 1/2[true positive/ (true positive + false negative) + true negative/ (true negative + false positive)] is the definition of balanced accuracy. It is also equal to (sensitivity + specificity)/2.
The area under the curve (AUC) of a receiver operating characteristic (ROC) curve shows the sensitivity against specificity at different thresholds (Table 1). The AUC-ROC has shown to be a helpful statistic for binary classifiers trained on unbalanced data sets (containing minority and majority classes). The kappa index is used to evaluate binary classification models for quality. The range of the kappa index is 0 (less significant) to 1 (perfect) [11].
Table 1. Cross-validation results for newly included models in the ProTox-II platform in terms of balanced accuracy, AUC–ROC, kappa value, sensitivity, and specificity. |
||||||
Items |
Models |
Balanced accuracy (%) |
AUC–ROC |
Kappa |
Sensitivity (%) |
Specificity (%) |
Organ toxicity |
DILI |
82.00 |
0.86 |
0.69 |
75.00 |
89.00 |
Toxicity endpoints |
Mutagenicity |
84.00 |
0.90 |
0.70 |
83.00 |
85.00 |
Carcinogenicity |
81.24 |
0.85 |
0.69 |
80.00 |
81.00 |
|
Cytotoxicity |
85.00 |
0.89 |
0.65 |
92.00 |
78.00 |
|
Immunotoxicity |
75.00 |
0.76 |
0.35 |
69.50 |
79.50 |
|
Toxicological pathways |
nr-ahr |
91.00 |
0.89 |
0.80 |
87.00 |
94.00 |
nr-ar |
93.00 |
0.84 |
0.75 |
89.00 |
97.00 |
|
nr-ar-lbd |
89.00 |
0.87 |
0.76 |
79.50 |
97.00 |
|
nr-aromatase |
92.00 |
0.86 |
0.79 |
78.00 |
96.00 |
|
nr-er |
90.00 |
0.75 |
0.71 |
85.00 |
95.00 |
|
nr-er-lbd |
89.00 |
0.85 |
0.73 |
83.00 |
95.00 |
|
nr-ppar-gamma |
92.00 |
0.81 |
0.71 |
86.00 |
97.00 |
|
sr-are |
91.00 |
0.84 |
0.69 |
85.00 |
97.00 |
ProTox-II is an easy-to-use platform that uses a variety of inputs to forecast chemical risk. Its output gives quick information on toxicity, including levels, approximate fatal doses, and comparable substances. The website provides access to comprehensive model information. The procedures, which are fully described on the ProTox-II website, consist of five steps that cover various toxicity concerns.
ProTox-II's future goals include improving its methods through the use of newly developed knowledge networks and genetic variances among people, guaranteeing accuracy in identifying harmful effects. Periodic updates, scheduled every three months, will include new information and endpoints, such as forecasts for genotoxicity, nephrotoxicity, neurotoxicity, cardiotoxicity, and food allergies, improving its usefulness for drug development and regulatory decision-making.
Continued advancements in ProTox-II aim to revolutionize toxicological evaluations further. By integrating newly developed knowledge networks and accounting for genetic variances among individuals, ProTox-II ensures greater accuracy in identifying potential harmful effects. Scheduled updates, occurring every three months, will incorporate additional information and endpoints, such as genotoxicity, nephrotoxicity, neurotoxicity, cardiotoxicity, and food allergies. These expansions will augment ProTox-II's efficacy in aiding regulatory decision-making and facilitating drug development processes. With its commitment to ongoing improvement and adaptation to emerging scientific insights, ProTox-II remains at the forefront of computational toxicology, poised to meet the evolving needs of the pharmaceutical industry and regulatory agencies.
To sum up, ProTox-II is a crucial computational tool that has the potential to revolutionize toxicological evaluations by providing thorough predictions over a wide variety of toxicity endpoints. It is also constantly changing to take into account new data and scientific discoveries [12, 13].
We would like to express our gratitude to the Principal and our Chairman Dr. Nalla G.Palaniswamy and Dr. Thavamani.D D Palaniswamy, Trustee, Dr. N.G.P Research and Educational Trust Coimbatore, for their guidance and support.
Ethics approval
This study does not involve experiments on animals or human subjects.
Data availability
All data generated and analyzed are included in this research article.
Funding
There is no funding to report.
Authors’ contribution
All authors have contributed equally.
Competing interests
The authors declare that they have no conflict of interest.
- Lea IA, Gong H, Paleja A, Rashid A, Fostel J: CEBS: a comprehensive annotated database of toxicological data. Nucleic Acids Res 2017, 45(D1): D964-D971.
- Thakkar S, Chen M, Fang H, Liu Z, Roberts R, Tong W: The Liver Toxicity Knowledge Base (LKTB) and drug-induced liver injury (DILI) classification for assessment of human liver injury. Expert Rev Gastroenterol Hepatol 2018, 12(1): 31-38.
- Huang R, Xia MH, Nguyen DT, Zhao T, Sakamuru S, Zhao J, Shahane SA, Rossoshek A, Simeonov A: Tox21 challenge to build predictive models of nuclear receptor and stress response pathways as mediated by exposure to environmental chemicals and drugs. Front Environ Sci 2017, 3: 85.
- Mayr A, Klambauer G, Unterthiner T, Hochreiter S: DeepTox: toxicity prediction using deep learning. Front Environ Sci 2017, 3: 80.
- Banerjee P, Siramshetty VB, Drwal MN, Preissner R: Computational methods for prediction of in vitro effects of new chemical structures. J Cheminformatics 2018, 8: 51.
- Siramshetty VB, Nickel J, Omieczynski C, Gohlke BO, Drwal MN, Preissner R: WITHDRAWN--a resource for withdrawn and discontinued drugs. Nucleic Acids Res 2016, 44(D1): D1080-1086.
- Liu J, Mansouri K, Judson RS, Martin MT, Hong H, Chen M, Xu X, Thomas RS, Shah I: Predicting hepatotoxicity using ToxCast in vitro bioactivity and chemical structure. Chem Res Toxicol 2015, 28(4): 738-751.
- Hansen K, Mika S, Schroeter T, Sutter A, ter Laak A, Steger-Hartmann T, Heinrich N, Müller KR: Benchmark data set for in silico prediction of Ames mutagenicity. J Chem Inf Model 2009, 49(9): 2077-2081.
- Schrey AK, Nickel-Seeber J, Drwal MN, Zwicker P, Schultze N, Haertel B, Preissner R: Computational prediction of immune cell cytotoxicity. Food Chem Toxicol 2017, 107(Pt A): 150-166.
- Huang R, Xia M, Sakamuru S, Zhao J, Shahane SA, Attene-Ramos M, Zhao T, Austin CP, Simeonov A: Modelling the Tox21 10 K chemical profiles for in vivo toxicity prediction and mechanism characterization. Nat Commun 2016, 7: 10425.
- Ahmed J, Worth CL, Thaben P, Matzig C, Blasse C, Dunkel M, Preissner R: FragmentStore--a comprehensive database of fragments linking metabolites, toxic molecules and drugs. Nucleic Acids Res 2011, 39(Database issue): D1049-D1054.
- Desbonnet L, Tighe O, Karayiorgou M, Gogos JA, Waddington JL, O'Tuathaigh CM: Physiological and behavioural responsivity to stress and anxiogenic stimuli in COMT-deficient mice. Behav Brain Res 2012, 228(2): 351-358.
- Richard AM, Williams CR: Distributed structure-searchable toxicity (DSSTox) public database network: a proposal. Mutat Res 2002, 499(1): 27-52.
- Cao B, Wang Y, Wen D, Liu W, Wang J, Fan G, Ruan L, Song B, Cai Y, Wei M, et al: A trial of lopinavir-ritonavir in adults hospitalized with severe covid-19. N Engl J Med 2020, 382(19): 1787-1799.
- Benfenati E, Manganaro A, Gini G: VEGA-QSAR: AI inside a platform for predictive toxicology. In CEUR Workshop Proceedings, CEUR-WS, 2020, 1107: 21-28.
Asia-Pacific Journal of Pharmacotherapy & Toxicology
p-ISSN: 2788-6840
e-ISSN: 2788-6859
Copyright © Asia Pac J Pharmacother Toxicol. This work is licensed under a Creative Commons Attribution-NonCommercial-No Derivatives 4.0 International (CC BY-NC-ND 4.0) License.